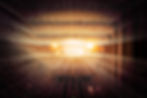
Reflections from the webinar
Why we held the event
“We need a more open, honest and humble exploration that involves the community.” Lela Kogbara. Director Global Black Thrive
In the process of setting up Place Matters we conducted research into the barriers and enablers of place-based change looking both at UK and international experience. We saw a particular difference in the way practitioners in countries with first nation communities and a diverse population gave prominence to acknowledging and managing power dynamics in place-based collaborations. Talking to people in Canada, Australia and New Zealand, practitioners had embedded an ethos and method around understanding and exercising personal power and privilege. We wanted to explore how UK practitioners were addressing unequal power dynamics and the various ways that inequality manifests itself.
Black Thrive Global are a practitioner partner of Place Matters and an organisation whose core purpose is tackling anti-Black racism in places. They have recently invested in establishing a research institute and observatory on racial equity, exploring how data and research can inform how we achieve racial equity. In this session we wanted to talk to the Black Thrive team about where they are in their journey to understand racial justice through research and data and how practitioners more widely can use data to better understand their response to racial inequity.
What we heard from Black Thrive
Dr Celestin Okoroji – Research Lead at Black Thrive talked first about how we imagine race and respond to racial differences illustrated by recent employment data in Lambeth. These “race imaginaries” illustrate the ways in people understand what race is and how such understandings connect to the ways we rationalise racial difference:
• Race as biology: Black people have different ‘genetics’ that means they are capable of different things than white people
• Race as culture: Black people have different traits and personalities that mean they are better suited to different things than white people
• Race as a social construct: we recognise that racial differences are artificial and understand that race differences flow from structural inequalities
• Race as “box ticking”: we pay lip service to race equity through procedures and polices without any genuine intent to be more equal and inclusive.
Understanding how we think about race as people and organisations is important to frame how we seek to address racial injustice.
Celestin gave examples of where employment and health data showed differences between Black and White people, but where it is not possible to investigate the data behind that headline difference. Such data only allows us to describe differences but not why those differences exist. This lack of transparency and detail perpetuates the sense that the difference is caused by race, rather than racism itself..
Dr Jolyon Miles-Wilson - Quantitative Research Fellow - talked about the homogenisation of racial identity for non-white people and the dominant premise of white people as the “norm” against which racialised groups are compared. Jolyon pointed out that the asymmetry in racialisation (i.e., attributing race to Black people but not white people) and homogenisation (i.e., assuming there is variation to explain within white populations but not in Black populations) has important implications for the way we design studies and analyse data. For example, homogenising terms can erroneously lead us to assume that there is little variation within and between Black populations, and data is consequently collected and analysed in a way that does not allow for adequate exploration of the experiences of individuals that are categorised in this way. Jolyon also talked about the notion of whiteness as a baseline against which other groups are compared. A product of this common implicit assumption is that the perspective we adopt to explain differences tends to center whiteness as normal and frames the Black experience as a deviation from normality. Jolyon highlighted that the framing we adopt can influence the questions we ask of data and in turn the utility of the answers we get from them. E.g. focusing on the extent to which white people deviate from a cohort average either positively or negatively.
Reflections from the discussions
Below are some of the points raised in the Q&A and the smaller groups discussion that followed.
Revealing the intersections with racial equity
Many organisations, including the NHS are on a journey to understand the intersections between health and the wider experience of people. Sharing data between organisations helps reveal the intersections that are less well understood or accessible through single data sources.
Analysis of data by racial difference
Analysis of data by race has the paradoxical purpose of continuing to reinforce false paradigms around racial difference, but whilst inequality persists, we must be able to see those inequalities clearly by looking at racial difference. The Black Thrive team talked about their internal debates around this paradox and how that illustrates the ethical complexity of the issues.
Visibility on the underlying causes of difference
Good quality person-level data allows us to eliminate (statistically) the differences that might account for inequality between people, revealing where racism and inequity is likely to be the underlying cause. In that sense access to good quality data at a level that allows deep analysis is a matter of social justice and organisations can contribute to tackling inequity by improving the capture and curation of data.
Reaching beyond quantitative sources
Data at a level that enables analysis within the Black population can highlight differences that are complex to understand and which require a broader consideration of the experience of people, their cultural backgrounds and perhaps the historical situations and expectations of families coming to the country. e.g. why children of African origin parents are high educational achievers compared to children of Afro-Caribbean parents. It is important that the level of data disaggregation allows us to understand the differences of experience between parts of the population so these deeper questions can be asked (and answered over time).
Capturing the voice and experience of Black people
Black people often make up a small sample size in a dataset and it can be difficult to access and understand the different parts of the Black community in an inclusive and representative way. Black Thrive talked about finding those voices to improve the representation and influence of Black people in general research.
Understanding why we think about race
As James Baldwin asked several decades ago, we must ask what society and those that define the data we use to make decisions want to achieve from the categorisation we use when we accept that those categories have become progressively reductive and negative? That we have not moved to a way of using data that properly captures the diversity and difference of people speaks to our wider attitudes towards creating equality of understanding and how prepared the system is to adapt.
Read, Watch, Do
The Black Thrive team has suggested some resources you can access to deepen your understanding.
Read:
The fire next time - James Baldwin
For white people getting to grips with race and racial justice, here is a list of eye-opening books that Jolyon found helpful:
So you want to talk about race - Ijeoma Oluo
Why I’m no longer talking to white people about race - Reni Eddo-Lodge
What white people can do next - Emma Dabiri
White Fragility - Robin DiAngelo
Watch:
Peter Hegarty: re-imagining group differences in scientific psychology
Do:
Let us know if you are interested in joining a workshop programme to dig deeper into questions about tackling racial inequity and power dynamics in place-based work. info@placematters.co.uk
Get in touch with Black Thrive to talk about collecting better data and analysing it with a racial justice lens: research@blackthrive.org
Explore and follow Black Thrive’s Research outputs on Github: https://github.com/BlackThrive
Register for Black Thrive’s next webinar ‘Changing the system: embedding lived experience in community grant-making’